As audience signal declines, the value of content is unlocked by “better contextual” that will alter the balance between the data-rich vs. the data-poor.
An advertising impression, whether displayed on the big screen, mobile, OOH etc., is a point-in-time relationship between three available levers: the 1) audience, the 2) creative content, and the 3) media content. When the three intersect successfully, the result is an ad impression that has an opportunity to capture attention and deliver the desired result. Through recent developments from Google, audience signals appears to be on the precipice of significant declining and is emerging as the hardest and weakest lever to pull.
Google’s Topics announcement earlier this year marked a shift away from Google’s FLoC proposal in favor of Topics. The announcement created a whole new round of challenges for marketers to activate audiences. While a great deal of confusion remains, Google’s announcement appears to have settled the uncertainty of how behavioral audiences will work in Chrome.
The consensus amongst AdTech commentators is that the signals provided by Google’s Topics are so “course” that the best alternative is to turn to” better contextual”. Also, between the lines in those comments, it is an admission that the AdTech industry has only ever focused on audience optimization and that the other aspects of the ad experience are not getting their share of attention.
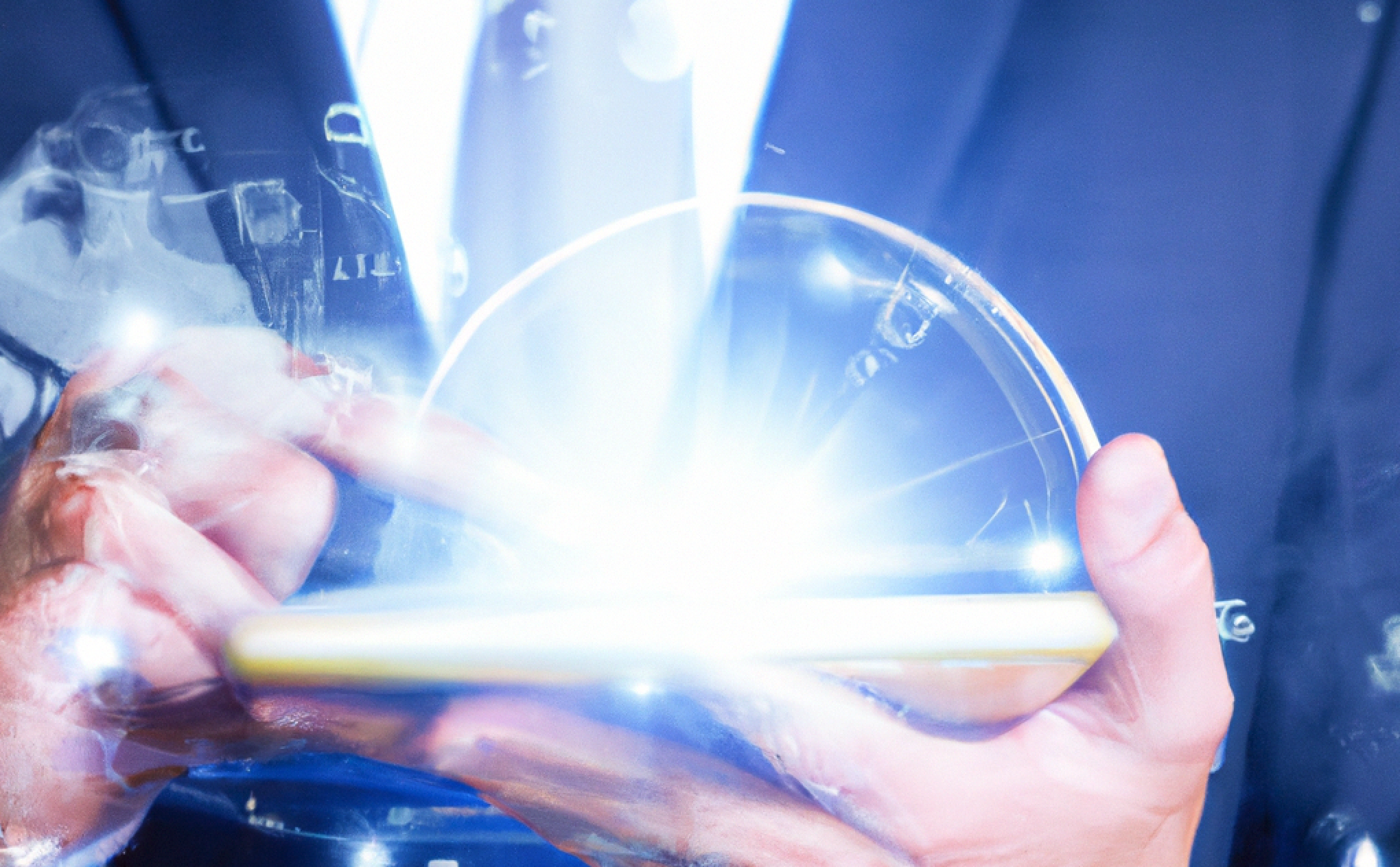
If “better contextual” is indeed the answer, then what is it?
With the well-known external factors such as the death of the 3P cookie and privacy regulations, addressable audiences are becoming harder to accumulate and activate at scale. If audiences are out of the picture, then there are still two other dimensions of the ad impression that can be optimized: the creative content and the media content. Media content on web browsers and to a lesser extent in CTV via companies like Iris.tv are what people think of when they hear contextual targeting. However, considering creative as the other ⅓ of the relevancy equation it has largely been unaddressed by AdTech.
With video (both in ad creative and media) quickly becoming the dominant form of human communication, creating a structured understanding of content so that can be used in optimization and modeling becomes the first-order opportunity for AdTech and the media industry. This opportunity has not been available before because image and video content data is too unstructured to use in modeling. However, the opportunity to structure image, video and text into a single taxonomy is enabled by recent advancements in the commercial viability of Computer Vision (CV).
The difference between text-first solutions and CV-enabled video-first solutions is that the text approach attempts to address image and video content by extracting text or metadata that is around the video or image and it avoids using the video and images themselves. This is mostly due to historic processing costs and legacy approaches for image and video vs. text but that has changed within the last year.
The text-first approach also does not work in the fastest growing and text-deprived area of content growth which is from short-form video that is distributed on platforms like TikTok, YouTube, Snap, and Meta. In the classification industry this is widely known as the “TikTok problem”.
The opposite side of to text-first solutions are video-first solutions that tackle the hardest classification problems first and then apply the methodology backward to image and text so all content has a consistent and structured classification that is the same across video, images, text in forms or URLs, text documents, and image and video formats like .jpg and .mov.
A video-first approach covers all format types so it can be effectively used by data science teams in modeling and optimization across creative and media content. Video first (vs. text first) solutions that achieve a comprehensive total content taxonomy is the definition of “better contextual”.
Computer Vision changes the game for who is data-rich vs. data-poor.
The shift from text-based solutions to video-first total content comprehension will also change the dynamics of the data-rich vs. the data-poor.
With Google’s shift, Adtech lost a huge chunk of reliable insights. But CRM data, authentication, and synthetic IDs no longer need to be the foundational footings of AdTech. CV can bridge the gap.
With CV, all activated content can be added to the shrinking geometric plane of data signal. Any reliable information from the activation, even if less precise than an identity- first solution like PII and synthetic IDs, are usable feedback for modeling and optimization that can cast a wider net than audience-dependent solutions. The reduced data sets that are ID dependent become more robust when structured signals from all content are added to the data plane and can feed optimization and analytics models.
Where we are headed.
Even without large sets of PII, directional personalization can be achieved at the aggregate level of a cohort if the multiple dimensions of the cohort like creative, audience signal, media content, time, etc. can be controlled. Cohort-s based solutions are also not dependent on PII because they work by triangulating aggregate data that is free from PII. PII can still be used as long ias it is aggregated into a cohort data model - but it is not a dependency. Instead, it plays the role of a sample or a “big panel”.
As an industry, the next wave of innovation moves from ID as a proxy for audience tracking to privacy-centric marketing through the effective implementation of cohort measurement and optimization models that are made more powerful through consistent comprehension across all content formats.
Interested in learning more? Click here to find a time to chat.